Harrison Huibin Zhou
Harrison Huibin Zhou
|
Ph.D. (2004) Cornell University
|
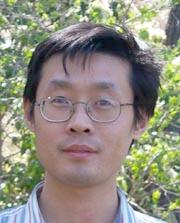
First Position
Dissertation
Advisor:
Research Area:
Abstract: Part I: Many statistical practices involve choosing between a full model and reduced models where some coefficients are reduced to zero. Data were used to select a model with estimated coefficients. Is it possible to do so and still come up with an estimator always better than the traditional estimator based on the full model? The James--Stein estimator is such an estimator, having a property called minimaxity. However, the estimator considers only one reduced model, namely the origin. Hence it reduces no coefficient estimator to zero or every coefficient estimator to zero. In many applications including wavelet analysis, what should be more desirable is to reduce to zero only the estimators smaller than a threshold.
We construct such minimax estimators which perform thresholding. We apply our recommended estimator to the wavelet analysis and show that it performs the best among the well--known estimator aiming simultaneously at estimation and model selection. Some of our estimators are also shown to be asymptotically optimal. This part is a joint work with J.T. Gene Hwang, which is to appear in the Annals of Statistics.
Part II: One of the most important statistical contributions of Lucien Le Cam is
the asymptotic equivalence theory. A basic principle of asymptotic equivalence theory is to approximate general statistical models by simple ones. A breakthrough of this theory was obtained by Nussbaum (1996) following the work of Brown and Low (1996). Nussbaum (1996) established the global asymptotic equivalence of the white-noise problem to the nonparametric density problem. The significance of asymptotic equivalence is that all asymptotically optimal statistical procedures can be carried over from one problem to the other when the loss function is bounded.
In this paper we established the asymptotic equvalence between the Gaussian variance regression problem and the Gaussian white noise problem under the Besov smoothness constraints. A multiresolution coupling methodology for the likelihood ratios (similar to the Hungarian construction) is used to establish asymptotic equivalence. For each resolution, our coupling approach is more elegant than the traditional quantile coupling methods; essentially we use quantile couplings between independent Beta's and independent normals. For the quantile coupling between a Beta random variable and a normal random variable, we establish a bound which improves the classical bound in KMT paper with a rate, which is of independent interest.
This is a joint work with Michael Nussbaum.